Business Transformation, Automation and Data Analytics Consultants
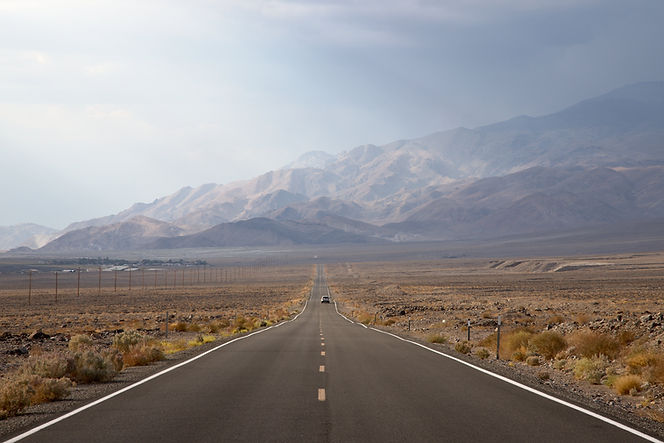
The Benefits of Institutionalizing Your Expertise
Creating a data-centric approach to decision making is a great way of institutionalizing the expertise that is held in the heads of the company's staff. The knowledge now becomes property of the company and is accessible by everyone who should have access to it. The company staff as a whole can benefit from this knowledge and everyone can contribute to further building of this institutional knowledge. It also ensures long term survivability of the company.
Oct 25, 2023
Background
​
There are many companies that last for many generations, but the majority of companies do not. There are many factors that affect the longevity of companies, but one very important factor is the ability to institutionalize knowledge in the company. In many SMEs, decision-making responsibilities are frequently concentrated in the hands of a very small number of people (often the business owner and/or other family members.) Furthermore, many of the factors that go into the decision making are kept in the mind of the decision-maker rather than being openly discussed or recorded. For example, the SME's purchasing manager decides to buy a large amount of oil because he thinks that prices have bottomed, that decision is often taken alone and not documented. Whatever goes on in his head at the time of decision-making stays in his head.
​
​
Who should not bother with this article?
​
Do not bother with reading this article if you are a business owner of an SME with the following business objectives:
-
No desire to scale the business - happy to remain a small business for the rest of your active working life.
-
No desire to extend the business beyond your personal retirement - when you choose to stop working, you will close the company down.
-
No desire to delegate any meaningful responsibility to your team. You insist on handling all major decisions yourself and will remain that way till the company is closed.
-
No desire to improve upon the efficiency of your decision-making process or the accuracy of your decisions.
-
Nobody on your team has any knowledge or expertise worth retaining in the company, or teaching others on the team.
​
If you and your team fit the description above, then there is nothing much in this article that will help your business.
​
​
Who will benefit from reading this article?
On the other hand, this article might be very helpful for you if you and your team have the following objectives:
-
A strong desire to scale the business - you want to keep growing the business, going from small to medium to large one day.
-
A strong desire to create a company that can continue operating even when you are no longer personally involved in the daily operations, such as when you retire.
-
A desire to delegate meaningful responsibility to your team, and to train your team up.
-
A strong desire to improve upon the efficiency of your decision-making process or the accuracy of your decisions.
-
A strong desire to retain the knowledge or expertise possessed by individual team members, and to spread that knowledge among the team members.
​
This article is only useful if your objective is to keep growing the company as much as possible, to create a structure that allows the company to continue operating even after you have stepped aside. This lays the foundation for successful enterprises that far outlive the founder.
​
​
Institutional Knowledge, Key Man Risk and the Further Institutionalization of Knowledge
​
Every successful company has a set of important institutional knowledge. Institutional knowledge is the entire set of experience, data, best practices, and other information that company employees use at work. This knowledge is used in the daily operations of the firm, whenever the team makes a decision or carries out a business-related activity. The knowledge is either generated during the work process or brought into the firm by new employees whenever they join.
​
Most SMEs do not have any rigorous data analytics process in place and often rely on two things:
-
The knowledge and ability of the particular individual making the decision. However, this knowledge and ability is owned totally by the individual and when he leaves, all these also leave. The firm retains very little of that ability and knowledge.
-
Luck and/or rule-of-thumbs. Do not bother with performing any complex decision making; just either rely on a lucky call or an arbitrary set of rules-of-thumb. The result is not optimized in any way, but who cares?
​
For example, in a fertilizer manufacturer, the procurement manager has to frequently purchase the commodity inputs required for the manufacturing process. He has to decide on when to purchase and how much to buy. There are a large number of variables to consider, such as expected future prices of the commodity, overall commodity market supply and demand trends, short-term fertilizer quantities expected to be manufactured for immediate sales, medium term buffer stock inventory levels of fertilizer required etc. If properly done, this is actually a complex dynamic optimization problem under uncertainty.
​
Most SMEs do not have such a sophisticated data analytics process in place, so we very often see one of two following possibilities appearing:
-
The Genius: The procurement manager happens to be a genius and is able to solve a simplified (but sufficiently effective) version of the optimization problem. He only considers a subset of the total set of variables that he should have considered and makes various approximations to reach the final solution. It is a 'good enough' solution.
-
The Go-by-Smell: The procurement manager simply does not bother with optimizing anything, and simply chooses the simplest way out regardless of the accuracy or optimality of that decision. For example, he just arbitrarily decides on what he thinks future prices of the commodity will be (without really analyzing any relevant industry or economic variables), makes the purchasing decision and goes to have lunch. He does not ever do any reviews on the accuracy of his past predictions. He keeps making the same biases and errors over the long course of his 20-year employment with the firm. (I call this the 'go-by-smell' approach because this is no different from making complex decisions based on what you are smelling at the point in time.)
​
Clearly, from the point of the firm, neither of these outcomes are ideal:
-
The Genius may leave the firm or retire one day. Because his thought process is largely in his head, his successor will not have access to it. The firm may end up with an inferior decision-making process because his successor, unfortunately, is not a genius like the original manager was. In other words, the original 'good enough' process is not replicable.
-
The Go-by-Smell is essentially trying his luck every time he makes a purchase. The firm has to bear the consequences of his luck. I think it goes without saying that it is not idea for a firm to have its raw material purchasing decisions be made based on luck.
​
The Genius is a clear example of "Key Man Risk". When the Key Man leaves, he takes all this knowledge with him and the company is unable to function as effectively as before because an important part of the institutional knowledge is lost to the company.
​
The best solution for companies is to keep adding to this institutional knowledge, further institutionalizing knowledge held by individual employees.
​
Since the advent of modern computers and databases, there has been a long tradition of institutionalizing this knowledge. For example, a Customer Relationship Management (CRM) software institutionalizes a large part of the knowledge held in the sales executive's head - identity and contact information of customers, past orders placed, and a host of other information pertaining to the client. Once this information is entered into the CRM, the company now owns a large part of the information that the sales executive used to monopolize and it becomes the company's asset. When the executive leaves, a large part of what he knows is retained by the company. The Key Man Risk is substantially reduced.
​
The benefits of institutionalizing knowledge are:
-
Reduce Key Man Risk
-
Allows the retention and sharing of knowledge among the team, which may lead to further improvements in the knowledge and skill levels of everyone on the team.
Allows the team to contribute and build upon the existing body of knowledge
Allows efficient sharing of knowledge among the team
Reduces Key Man Risk
How a Data-driven approach helps the institutionalization of knowledge?
​
A data-driven approach to running the business substantially increases the ability and need to institutionalize knowledge. The data-collection and data-analysis steps will result in the institutionalization of knowledge - data and analysis will be transferred from the employees to the company (store in the database).
​
The modern data-driven approach allows us to institutionalize knowledge across a far wider range of topics and cover all aspects of a company's daily operations. We can have databases covering many aspects of a company's operations - internal administration, client-facing, factory production etc.
​
​
Example: Factory operations
In many SMEs, factory production lines are not monitored or calibrated as carefully as in large companies like TSMC. At a large semiconductor fab like TSMC, every tiny step in the chip production process is carefully monitored, data collected and analyzed, detailed recipes are created and everything is done by the book. In many SME factories, not that much data is collected and there is a lot of leeway given to production line managers to tweak the production process 'based on experience'. There is no detailed experimentation to assess how different production process settings affect production yields. The 'experience' of the production line managers is kept in their heads. The assumptions and conclusions used by the production line managers in their decision making may or may not be correct, and we are often unable to test them. When an experienced production line manager leaves or retires, there is often a drop in production yields when a new less experienced manager takes over.
​
If SME factories follow TSMC's approach and adopt a data-driven approach, this production knowledge will be kept in the firm. When one manager leaves, the next manager can pick up where the earlier one left off. More important, he will be able to add to the existing information, and the body of useful information keeps increasing.
​
What can we do for the client?
​
In this example, we can collect and analyze the data from his production line to analyze the following:
-
Where are the bottlenecks?
-
Where are the sources of instability in the production line?
-
What are the possible areas that often cause problems, such as stoppages and low yields?
-
Discuss potential solutions with production line staff.
A useful outcome will be a set of 'recipes' for the production line which any production manager can implement and monitor.​
​
​
Example: Purchasing of commodity inputs
​
In many SMEs that require purchasing commodity inputs like oil and copper, the decision-making is done in a fairly arbitrary manner by a small handful of people. There is often no research team to analyze price trends and no notetaking to record the rationale behind any particular purchasing decision. There is almost no post-trade postmortem to analyze the accuracy of the particular purchasing decision. If the purchasing manager was effective and made good purchasing decisions, the firm does not have detailed records of the underlying process. When the existing purchasing manager leaves the firm, the next person to replace him may not have as good of a process and his decisions may not be as good. The firms loses.
​
More importantly, the firm will never be able to build upon the experiences of previous purchasing managers, and never be able to learn from the good and bad decisions made in the past. There is no institutional memory, no institutional learning.
​
On the contrary, if there is a data-driven approach that captures the entire decision-making process, the firm can retain that knowledge and constantly improve the process. The firm can review the inputs used, the analysis done and the conclusions drawn. With enough data, we can build statistical models and machine learning models to help in the prediction of the optimal timing and quantity of purchase.
​
What can we do for the client?
​
In this example, we can work with the client to develop a rigorous decision-making process that statistically analyzes the impact of the key variables on expected pricing. We can input these expectations into the commodity ordering model.
We begin by working with the existing processes currently being used and build upon these processes. Eventually, we will have a model that institutionalizes the knowledge of the existing commodity purchasers within the firm, and adds to that knowledge.
​
​
Conclusion
​
The adoption of a data-centric approach requires the following steps:
-
Creation of a database
-
Creation of a data analysis process
-
Interpretation of the results of the data analysis
-
Action (based on the Interpretation)
-
Postmortem review of the consequences of the Action
​
These steps effectively replicate a lot of the knowledge held by the individual and his decision-making. Once complete, we would have institutionalized a large portion of the information in the individuals' head. If the individual leaves the firm, the firm can continue operating as before with minimal disruption. This allows the firm to scale and continue beyond the working lifespan of any individual founder.
​